THANK YOU FOR SUBSCRIBING
Editor's Pick (1 - 4 of 8)

Big Data Analytics
Dr Li Xiaoli, Head of Data Analytics Department, Institute for Infocomm Research (I2R), A*Star

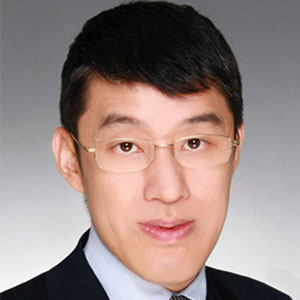
Dr Li Xiaoli, Head of Data Analytics Department, Institute for Infocomm Research (I2R), A*Star
Typically Rich Relevant Data Can Potentially Lead To More Useful Insights Or Accurate Prediction Models
Weekly Brief
I agree We use cookies on this website to enhance your user experience. By clicking any link on this page you are giving your consent for us to set cookies. More info
Read Also
The AI Rat Race - Keeping Up with New Technologies or Waiting for Maturity?
Andreas Kurz, Global Head of Digital Transformation, ALFAGOMMA Group
The Role Of Digitalization In Transforming Airport Customer Experiences
Kebebew Bulto, Project Manager, Ethiopian Airlines
From Chemical Solutions to Data Center Innovations
Akmal Zharif Bin Abdullah, Datacenter Manager, Aeon Credit Service (M) Berhad [KLSE: AEONCR]
Ethics & Compliance in a Digital World: Navigating HCP Engagement in APAC
Sherene Cham, Regional Director, Ethics & Compliance – APAC, Menarini Group
How AI is Driving Innovation and Customer-Centricity in Insurance
Xinfa Cai, Chief Innovation Officer, AIA Group
The Impact of Smart Infrastructure on Growing Cities
Scott Edward Sidhom, Manager Infrastructure Planning, Liverpool City Council
Transforming Engineering through Data Science
Agus Jamaludin, Data Scientist Lead, Petrosea
Redefining Risk Management: Strategies for a Safer Future
Nhu Nguyen, Risk Data Analysis Manager, Pnj Group
